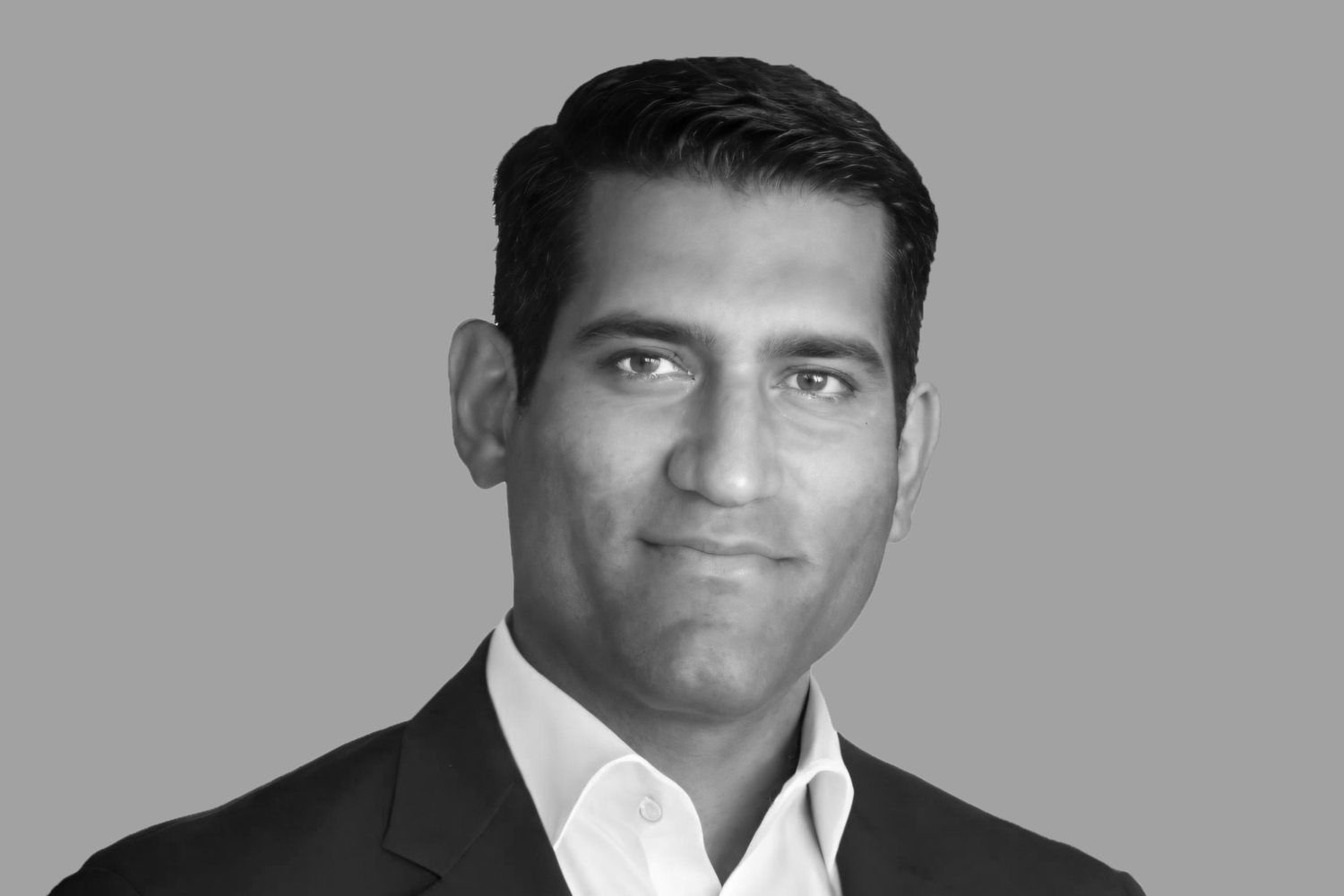
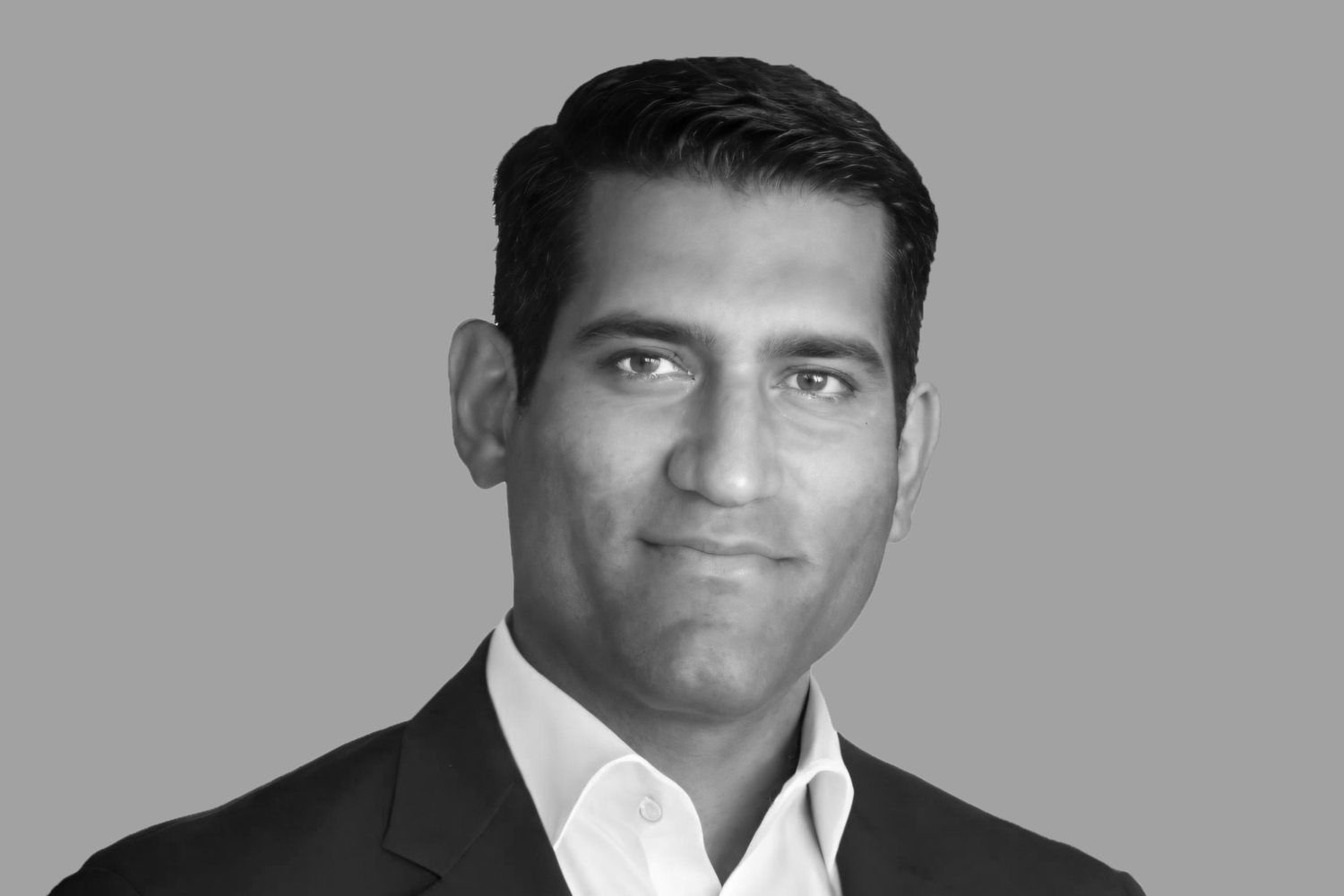


AI shows tremendous promise in healthcare, from improving patient care and operational efficiencies to early detection of disease and personalized treatment plans. The potential of AI in a patient-centered environment depends on understanding its capabilities, its limitations, and the essential role of human oversight. While AI can greatly enhance human capabilities, it is not infallible and cannot replace human expertise. Recognizing and preparing for the predictable (and unpredictable) outcomes of AI, ensuring responsible oversight, and promoting transparency are important criteria for effectively integrating AI into healthcare.
Applications of AI in health care is diversifieddelivering improvements in diagnosis, treatment planning, patient monitoring and administrative efficiency. For example, AI-based diagnostic tools analyze medical images to detect conditions such as cancer and cardiovascular disease, improving early detection and patient outcomes. Others, like Suki AI, integrate with a clinician’s EHR, generate notes, suggest codes, and perform dictation. AI-powered personalized care plans reduce adverse effects and improve treatment effectiveness. AI also continuously monitors patient data in real time, alerting healthcare providers to potential issues before they become critical. Additionally, AI streamlines administrative tasks such as scheduling and billing, reducing the burden on administrators.
Data integrity and diversity
One of the challenges of developing AI is the need to use both existing and new data. While existing datasets such as EMRs or clinical trial data are important, new data is essential for training robust AI models. Additionally, various bias-eliminating datasets are needed to influence the accuracy and reliability of AI results. This ensures that AI models can perform optimally, leading to more accurate diagnoses and effective treatments. Ensuring data integrity, completeness, and consistency is necessary to develop AI systems that healthcare providers can trust and rely on.
AI technology is already being used in many industries, including finance, manufacturing, and education. It is understood that AI is not flawless in these sectors. Just as we struggle with imperfections in everyday technologies, such as AI sensors in cars that can sometimes give false alarms due to glitches, we need to adopt a similar mindset for AI in the field of health. Recognizing that AI systems may have limitations or errors is important for effectively integrating them into healthcare workflows. Rather than expecting flawless performance every time, we should focus on developing integrated systems that are sufficiently transparent, explainable and reliable to help healthcare professionals make informed decisions and deliver highly personalized patient care. .
The probabilistic nature of AI
The probabilistic nature of AI means that it relies on inherently uncertain statistical models, sometimes compounded by biases in the training data. In healthcare, where errors can lead to incorrect diagnoses or ineffective treatments, this is particularly important. Strict accuracy standards and robust validation processes are necessary to minimize these risks. But even with all these safeguards, AI systems sometimes fail. Designing systems with predictable failures allows healthcare providers to create workflows and contingency measures to effectively manage these failures. Human monitoring can ensure accuracy and resolve any issues.
Intelligent decision support
Despite significant advances in healthcare practices over the past decades, human error remains a persistent challenge, particularly with regard to diagnostic delays or errors. The research report “To err is human. » highlighted this problem nearly 25 years ago, estimating that up to 98,000 people die each year due to medical errors in hospitals. In this environment where thousands of decisions are made every minute, AI-based decision support can aid and improve human decision-making processes. By using AI’s analytical capabilities, healthcare professionals can access data-driven insights, improve diagnostic accuracy, reduce errors, and ultimately improve patient outcomes. Integrating AI into healthcare workflows can complement human expertise, but also serves as a tool to address complex challenges associated with diagnostic errors and delays.
For successful implementation of AI in healthcare, building trust through transparency and explainability is a top priority. Seamless AI systems enable healthcare professionals to understand decision-making processes, verify results, and make informed decisions. Explainable AI models help professionals understand the reasoning behind AI recommendations, promoting trust and effective use.
Comprehensive training programs can equip healthcare professionals with the knowledge and skills to effectively use AI tools, covering technical aspects, ethical considerations and practical applications. Additionally, developing user-intuitive systems that solve the problems inherent in AI is important for success. By ensuring these systems are accessible and simple, we can facilitate seamless integration into daily workflows. Continuous education and training builds user confidence and helps create a collaborative environment where AI and human expertise come together to improve patient outcomes.
To successfully integrate it into healthcare, it is imperative to balance the promise of AI with a realistic understanding of its capabilities and the need for human oversight. Setting realistic expectations, leveraging AI’s strengths, and addressing its inherent uncertainties can improve the safety and effectiveness of AI applications. Harnessing its potential while maintaining a calculated and informed approach ensures that these technologies contribute positively to patient care and healthcare outcomes.
About Kabir Gulati
With 14 years of expertise in health technologies, Kabir Gulati joins Owner as vice president of data applications. Previously, Kabir was Vice President of Product, Design and Analytics at CancerIQ, where he led a team responsible for delivering software solutions for cancer prevention, driving efficiencies and improving provider-patient satisfaction.
Kabir excels in innovating and delivering impactful software solutions in healthcare technology, leveraging his extensive experience and strategic vision to improve operational efficiencies and provider-patient satisfaction. His expertise will play a crucial role in improving surgical navigation through data-driven solutions.